3D Study of TEG-based Energy Harvesters With Physics-informed Neural Networks
A fully-parameterized 3D model of thermoelectric generator (TEG)-based energy harvesters is built. With the use of a coupled CFD and heat transfer FEM simulation, the temperature field within the complete system (heat source, TEG, cooler, ambient air) is computed. Especially the temperature difference between the cold and warm side of the TEG is important to calculate the harvestable energy. Such an extensive multiphysical simulation takes several minutes to hours until the desired data (e.g. provided energy) is available for one set of input parameters (e.g. design of the cooler, properties of the TEG). In the next step, this FEM-based model is consequently used to generate data for a physics-informed neural network (PINN). A Latin hypercube algorithm is employed to generate ideal sample data for the training of two PINNs: One neural network is the one implemented in COMSOL and a second one is selfmade with Python. Ultimately, these two PINNs are compared with respect to their prediction accuracy and computing time advantage over the ‚full‘ FEM solution.
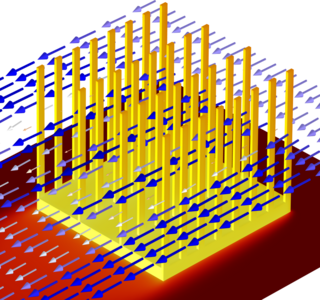
下载
- lohbreier_8901_poster.pdf - 0.48MB