Inverse Analysis of Soil Parameters Based on Deformation of a Bank Protection Structure
Deformation prediction is an important part of the structure stability analysis. However, the deformation of bank protection structure which is affected by many factors, such as structural stiffness, earth pressure and hydrostatic pressure. It contains a complex mechanical process, which is a grey, fuzzy, stochastic and nonlinear engineering problem[1]. Neural network method as one of nonlinear inversion methods, is based on a large number of measured data. It has a good prediction ability which is realized by establishing the nonlinear mapping between input and output[2-3]. However, this method does not involve mechanical mechanism of the deformation, and it is difficult to grasp the key factors on the deformation process. Thus, we try to use a joint inverse method to solve this problem. In this work, we present an inverse analysis combining numerical simulation method and neural network by using COMSOL Multiphysics®. Firstly, we establish a forward model to map the function of main soil mechanical parameters and the deformation results calculated by coupling the physics interfaces of Darcy’s Law and Solid Mechanics. Subsequently, based on MATLAB® neural network toolbox, the generalization ability of BP neural network model by using the LiveLink™ for MATLAB® applied in the deformation prediction of bank protection structure is verified by comparing the calculated values and measured values. Finally the optimal mechanical parameters of soil will be obtained by this joint inversion method. In the further study, we will estimate the reliability of this method by comparing numerical results using the inversed, predicted values by BPNN model, and the in-situ measured displacements.
Reference 1. Henrik Wall, Lars Wadso, Corrosion rate measurements in steel sheet pile walls in a marine environment, Marine Structure, vol.33, pp. 21-32, 2013. 2. M. A. Shahin, M. B. Jaksa, and H. R. Maier, “Artificial neural network applications in geotechnical engineering,” Australian Geomechanics, vol. 36, no. 1, pp. 49–62, 2001. 3. Z.-Z. Liang, B. Gong, C.-N. Tang et al., “Displacement back analysis for a high slope of the Dagangshan hydroelectric power station based on BP neural network and particle swarm optimization,” The Scientific World Journal, 2014.
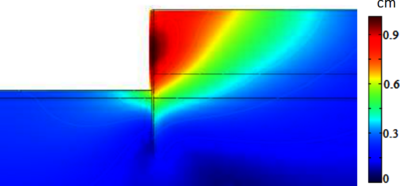
下载
- xing_poster.pdf- 0.48MB
- xing_paper.pdf- 0.58MB
- xing_abstract.pdf- 0.02MB