Neural Network Surrogates for Backward-Facing Step Flow up to Re=900 in COMSOL Multiphysics®
Over the past 10 years, scientific, technical, and technological advancements have significantly propelled the integration of artificial intelligence and machine learning technologies within the field of physics, particularly in numerical simulations and the design of autonomous and efficient Digital Twins. In 2023, COMSOL Multiphysics® introduced, for the first time, functionalities of Surrogate Models, including the ability to train non-informed neural networks using experimental data or models within the software. By training on data from velocity and pressure fields, we evaluated the accuracy and performance of these networks in predicting the backward-facing step steady-state flow for Reynolds numbers (Re) up to ~900, comparing the results to experimental data. We tested various configurations of these networks and the Laminar Flow CFD model samples generated to train them. We measured both the validation accuracy (e.g., for Re<580 and Re<400) and the generalization accuracy of the trained networks in Reynolds number and spatial domains. Our preliminary work indicates that the most optimized neural networks are able to predict the extension of the primary recirculation zone with a minimum validation accuracy of 0.5% and a generalization accuracy ranging between 5.8% and 14.4%. We demonstrated that a neural network trained on 2D numerical simulation data for Re < 400 - where results align within 5% of experimental data - is able to produce generalization predictions consistent with experimental results in the range of 400<Re<920, where the flow becomes three-dimensional. With a computing time of ~0.6 seconds, the neural networks are 12.5 to 14 times faster than a non-linear stationary PARDISO solver operating on the same mesh within COMSOL Multiphysics®.
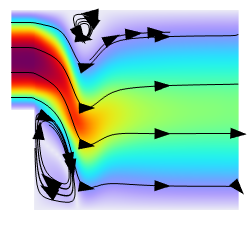