Using Surrogate Models to Predict the Transmission Efficiency of an ERS
Surrogate Models are used to predict the power transmission of an Electric Road System (ERS). The ERS consists of a transmitter coil integrated into the road and a receiver coil underneath an electric vehicle. Accordingly, this technique enables the dynamic charging of the vehicle via inductive power transfer (IPT). To enhance the transmission efficiency, a ferrite plate behind the receiver coils can be used to guide the magnetic field.
For the optimization of the ferrite core design, a multi-scale FEM model of the ERS is built using the AC/DC module of the simulation software COMSOL Multiphysics. The simulation domain consists of the transmitter DD-coil and the receiver DDQ-coils which are surrounded by an air domain (see Figure 1). The transmitter coil is excited by an alternating current (AC), which generates a time-harmonic magnetic field. For the proof of concept, the receiver coils are short-circuited, and the short-circuited current is used as a measure of the transmission efficiency. In addition, a ferrite plate is considered, which is centered above the receiver coils (see Figure 2).
The 3D FEM simulations of the ERS are conducted to generate training data for a deep neural network (DNN). In total, 6 parameters are varied to train the network, namely the dimensions of the ferrite plate (width, length, and thickness) and the relative positions of the transmitter and the receiver coils (the offset in x-, y-, and z-direction). As output parameters, the DNN predicts the mass of the ferrite plate and the currents in both receiver coils. The architecture of the neural network is sketched in Figure 3.
In this research, a proof of concept is performed to build a kind of a physics-informed neural network (PINN) by the combination of FEM simulations and DNN. After the training and validation phase, the PINN will be able to predict various parameters like the transmission efficiency and the weight of the ferrite core with much lower costs than a FEM simulation, but with comparable accuracy. However, if the reliability of this method is ensured, it is a powerful development approach to optimize even a free-shape ferrite core structure in future projects.
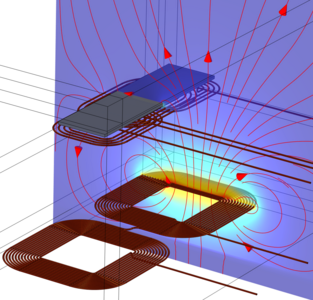
下载
- fromme-10781-poster.pdf - 1.64MB